According to a recent report by Experian, data quality issues had a detrimental effect on 95% of business leaders surveyed in 2021. The repercussions ranged from negative customer experiences to a loss of customer trust, underscoring the critical importance of data quality.
While collecting data is a crucial step, the true challenge lies in consistently upholding high standards of data quality throughout its entire lifecycle.
In today’s blog post, we will delve into the top ten data quality issues that many businesses face and explore some easy solutions.
Subscribe to Our Newsletter
Join Our Newsletter
Subscribe to our newsletter and receive the latest news and exclusive offers from Bill Moss Data.
1. Duplicate Records Data Quality Issue
Duplicate records refer to the existence of multiple instances of the same data within a dataset or database.
These duplicates can occur for multiple common reasons, such as data entry errors, system glitches, or merging of data from different sources. Duplicate records not only waste storage space but also lead to data inconsistency and hinder accurate analysis.
To fix duplicate data records, organisations can implement data deduplication processes. This involves identifying duplicate records through matching algorithms or comparison techniques and merging or removing redundant entries.
Automation tools, data cleaning, or data management software can all help aid in resolving duplicate records to ensure and improve quality.
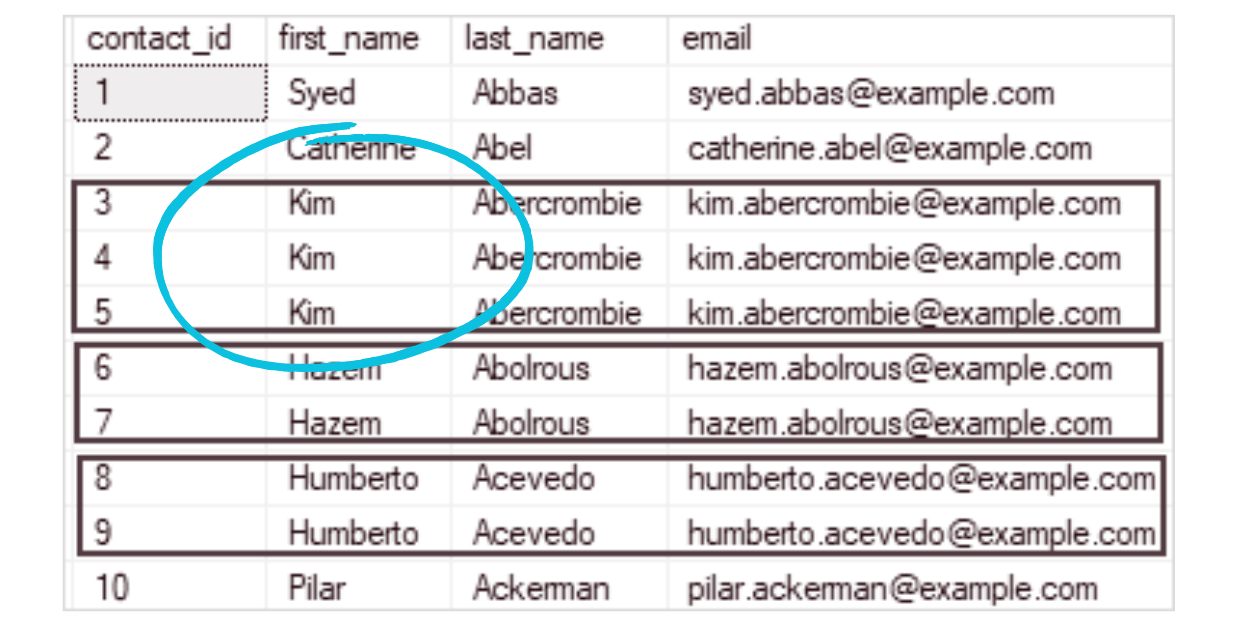
2. Incomplete Data Quality Issue
Incomplete records are data entries that lack necessary or essential information within a dataset or database. This type of error can occur anywhere from mistakes during data entry, data migration issues, or gaps in data collection processes. Entries that aren’t completed limit data accuracy which in turn impacts analysis capabilities.
In order to fix incomplete records, organisations can establish practices for regular data validation and updates. This involves conducting periodic data audits to identify incomplete records and proactively fill in missing information.
3. Inconsistent Formatting Quality Issue
Inconsistent record formats refer to the lack of standardisation in the structure, format, or representation of data within a dataset or database.
For example, inconsistent date formats like “MM/DD/YYYY” and “DD/MM/YYYY” can cause confusion and errors during data analysis. This can hinder data integration efforts, make data processing challenging.
To solve inconsistent record formats, organisations should establish and enforce data formatting standards. This involves defining a consistent format for each data attribute, such as dates, phone numbers, or addresses, and ensuring adherence across the dataset.
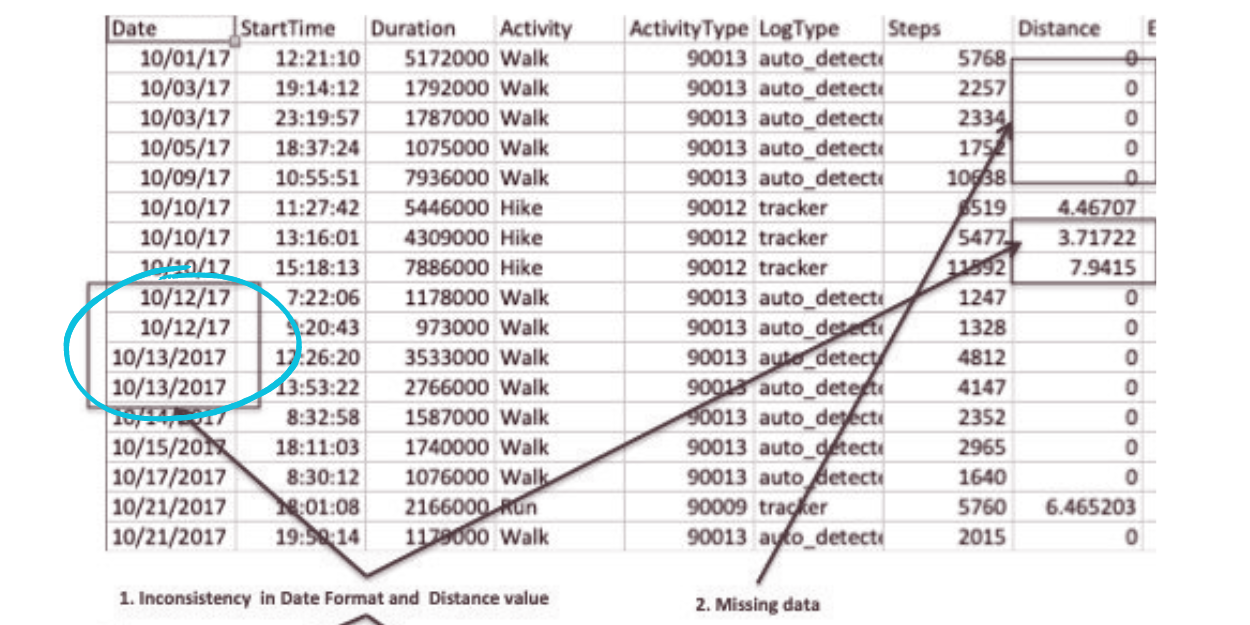
4. Inaccurate Data
Data inaccuracies can come from various sources, including manual errors during data entry or outdated information that is no longer valid.
To prevent data inaccuracy issues, organisations should prioritise regular data validation and verification checks as part of their data management practices. By implementing robust validation processes, such as automated validation rules or manual reviews, businesses can identify and rectify inaccuracies promptly. Additionally, leveraging data cleansing tools can help identify and correct common errors, such as misspellings, duplicates, or inconsistent formatting.
Establishing data governance practices, including data quality standards, data stewardship roles, and clear processes for data maintenance, further contributes to maintaining data accuracy.
5. Non-Standardized Data Quality Issue
A non-standardized data quality issue refers to data that lacks uniformity or adherence to a predefined set of standards within a dataset.
Let’s look at an example together. In an inventory management system, product sizes may be recorded inconsistently as “S,” “Small,” or “Size 4.” This inconsistency makes data integration and analysis challenging, hindering effective decision-making.
To solve problems with non-standardized data, organisations should establish and enforce data standards and conventions. This involves defining and implementing a consistent set of rules for data formatting, naming conventions, and classification systems. By normalising and standardising data across all relevant fields, organisations can ensure consistency and facilitate seamless data integration, analysis, and reporting.
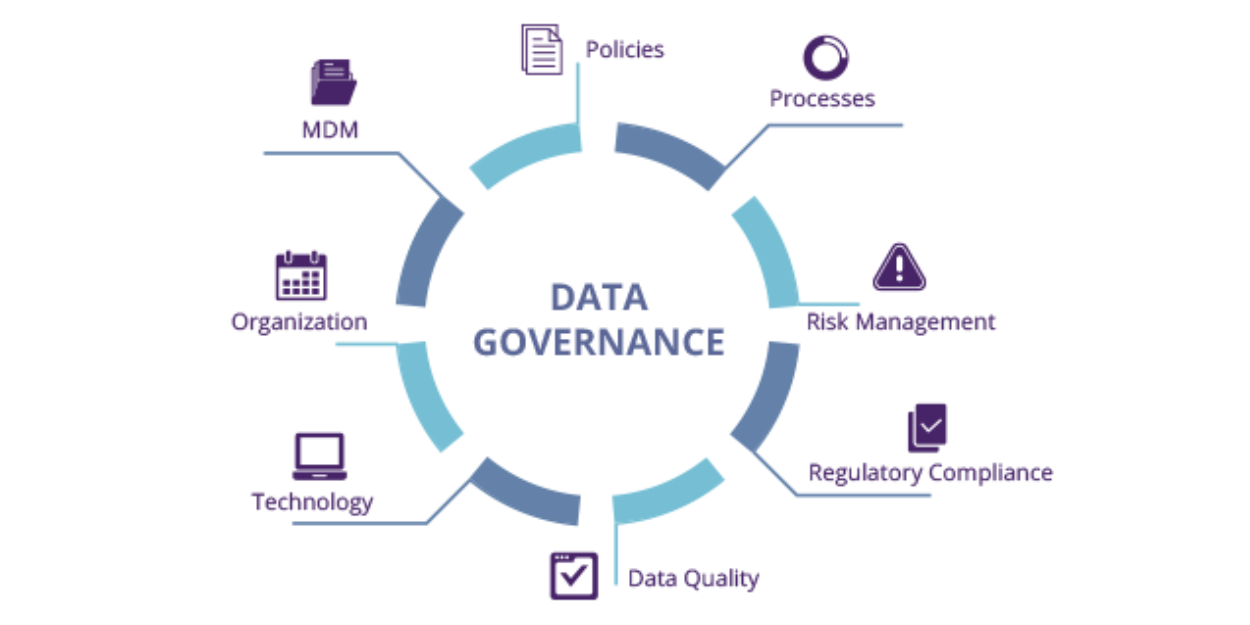
6. Lack of Data Governance
A lack of data governance is the absence or inadequate implementation of policies, processes, and controls to manage and govern data. Without proper data governance, organisations face challenges such as data inconsistencies, security risks, and lack of accountability.
For example, in a company with no data governance framework, different departments may have their own data storage systems and practices. This could in turn lead to data silos and inconsistencies across the organisation.
To address this, organisations should establish a robust data governance framework. This might include defining data ownership and responsibilities as well as implementing procedures for data quality and compliance.
7. Data Integration Challenges
Data integration issues may arise when data from multiple sources or systems cannot be seamlessly combined and consolidated due to differences in data formats.
For example, when merging customer data from CRM and ERP systems, inconsistent field names or varying data formats can hinder effective integration.
To address data quality and integration issues, organisations can use data cleansing. Data cleansing involves the identification and correction of errors, inconsistencies, and redundancies in the data.
By employing data cleansing tools and processes, businesses can standardise data formats, resolve naming discrepancies, eliminate duplicate or outdated records, and enhance data quality.
8. Data Decay
Over time, data becomes outdated, impacting its relevance and accuracy.
This naturally occurs over time as information becomes outdated and loses its accuracy due to changes in various outside factors.
To fix data decay issues, organisations can implement proactive measures. Firstly, establish processes for regular data monitoring and maintenance. This includes conducting periodic data audits to identify outdated or inaccurate information and updating or removing it accordingly.
Secondly, leverage data enrichment services or external data sources to append missing or outdated data.
Then last but not least, automate data updates to ensure data remains up-to-date in real-time. By addressing data decay through proactive data maintenance organisations can ensure the integrity and currency of their data.
9. Data Security and Privacy
Insufficient data security measures can have severe consequences in both data quality issues and customer trust. In an increasingly digital world, data breaches and unauthorised access to sensitive information pose significant risks.
To address this, organisations must prioritise strong security measures to protect their data assets. This includes implementing encryption techniques, firewalls, and secure authentication protocols to safeguard data from external threats.
Organisations should also adhere to relevant data privacy regulations, such as GDPR or CCPA, to ensure total compliance and protect customer privacy. Implementing strong data security measures and promoting a culture of data privacy can help mitigate risks and foster trust with both customers and stakeholders.
10. Poor Data Entry and Validation
Errors during data entry and insufficient validation processes can introduce inaccuracies into data sets, undermining data quality and decision-making.
To combat this, organisations should invest in training staff on proper data entry techniques. This training can cover guidelines for accurate and consistent data entry, including standardised formats, data validation requirements, and the importance of attention to detail.
Furthermore, implementing validation rules can help identify and prevent common errors, such as missing fields, invalid formats, or out-of-range values. Validation rules can be applied at the point of data entry or integrated into data management systems. Automating validation processes through data validation tools or software can further enhance efficiency and accuracy by automatically flagging potential errors or inconsistencies.
In Conclusion…
Addressing data quality issues is essential for organisations striving to make data-driven decisions and achieve their business objectives. By understanding and tackling the ten most common data quality challenges, organisations can unlock their data’s potential.
At Bill Moss Data, we understand the significance of data quality and offer comprehensive data solutions to assist organisations in overcoming these challenges. Visit www.bill-moss.com to explore our range of data services, including data cleansing, validation, and enrichment, designed to optimise your data quality and enhance your decision-making capabilities.
Related Articles
B2B Data: What it is and how to use it effectively
For B2B sales representatives, the quality and depth of data about a prospect can directly impact the effectiveness of their pitch. In 2023 data has become a driving force behind critical decision-making. Data provides invaluable insights to marketing and sales...
Data Cleansing: The Key to Accelerating Business Growth
Discover how data cleansing can transform your business processes and drive growth. In today's digital age, data is king. From customer information to sales data, businesses are constantly collecting vast amounts of data. However, as valuable as data is, it can also...
GDPR and B2B Data Management: A Game-Changer for Business Success
Discover how data cleansing can transform your business processes and drive growth. Data management is more important than ever in 2023. But with the advent of GDPR, managing data has become even more complex. For B2B companies operating in Ireland, GDPR has...